Özyeğin Üniversitesi, Çekmeköy Kampüsü Nişantepe Mahallesi Orman Sokak 34794 Çekmeköy İstanbul
Telefon : +90 (216) 564 90 00
Fax : +90 (216) 564 99 99
info@ozyegin.edu.tr
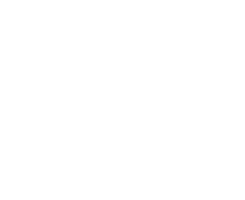
Thesis Defense - Furkan Arslan (MSCS)
Furkan Arslan – M.Sc. Computer Science
Asst. Prof. Reyhan Aydoğan– Advisor
Date: 27.08.2021
Time: 10:00
Location: This meeting will be held ONLINE. Please send an e-mail to gizem.bakir@ozyegin.edu.tr in order to participate in this defense.
Associative and Frequentist Opponent Modeling Approaches in Automated Bilateral Negotiations:
Thesis Committee:
Asst. Prof. Reyhan Aydoğan, Özyeğin University
Prof. Erhan Öztop, Özyeğin University
Asst. Prof. Emre Uğur, Boğaziçi University
Abstract:
Designing an effective and intelligent bidding strategy is one of the most compelling research challenges in automated negotiation, where software agents negotiate with each other to find a mutual agreement when there is a conflict of interests. Instead of designing a hand-crafted decision-making module, this work proposes a novel bidding strategy adopting an actor-critic reinforcement learning approach, which learns what to offer in a bilateral negotiation. An entropy reinforcement learning framework called Soft Actor-Critic (SAC) is applied to the bidding problem, and a self-play approach is employed to train the model determining the target utility of the coming offer based on previous offer exchanges and remaining time. Furthermore, an imitation learning approach called behavior cloning is adopted to speed up the learning process. Also, a novel reward function is introduced that does not only take the agent's own utility, but also the opponent's utility at the end of the negotiation. The developed agent is empirically evaluated. Thus, a large number of negotiation sessions are run against a variety of opponents selected in different domains varying in size and opposition. The agent's performance is compared with its opponents and the performance of the baseline agents negotiating with the same opponents. The empirical results show that our agent successfully negotiates against challenging opponents in different negotiation scenarios without requiring any former information about the opponent or domain in advance. Furthermore, it achieves better results than the baseline agents regarding the received utility at the end of the successful negotiations.
Bio:
Furkan Arslan was born in Erzincan. He was graduated from İstanbul Cagribey Anatolian High School in 2010. In June 2014, he received the degree of Bachelor of Science in Computer Engineering from Hacettepe University. In 2020, He founded a start-up named Counterfake. He is currently working as a CEO in this company.